I first learned about neurons from a lecture by physicist and now newly minted Nobel Prize winner John Hopfield at Bell Telephone Laboratories in 1985. Hopfield was a senior scientist there, while I was as junior as possible. Bell Labs — the inventors of the transistor — had sponsored a set of lectures focusing on Hopfield’s new mathematical discovery that physics equations could explain neural circuits.
In his lecture, Hopfield showed diagrams of networks now called “Hopfield networks.” Bell Labs was hosting a Hopfield network day honoring him and his new-fangled ideas of using relaxation-energy equations from physics to design networks that “solved” certain difficult problems. Some of his diagrams looked just like the operational amplifiers (op-amps) in electronic circuit diagrams, which I had tinkered with for years — but now he called them neurons. So, my skill at making circuits now applied to brains. I was hooked, and within three years I was accepted as a student in the brand-new academic program called Computation and Neural Systems (CNS) that Hopfield was founding at the California Institute of Technology (Caltech).
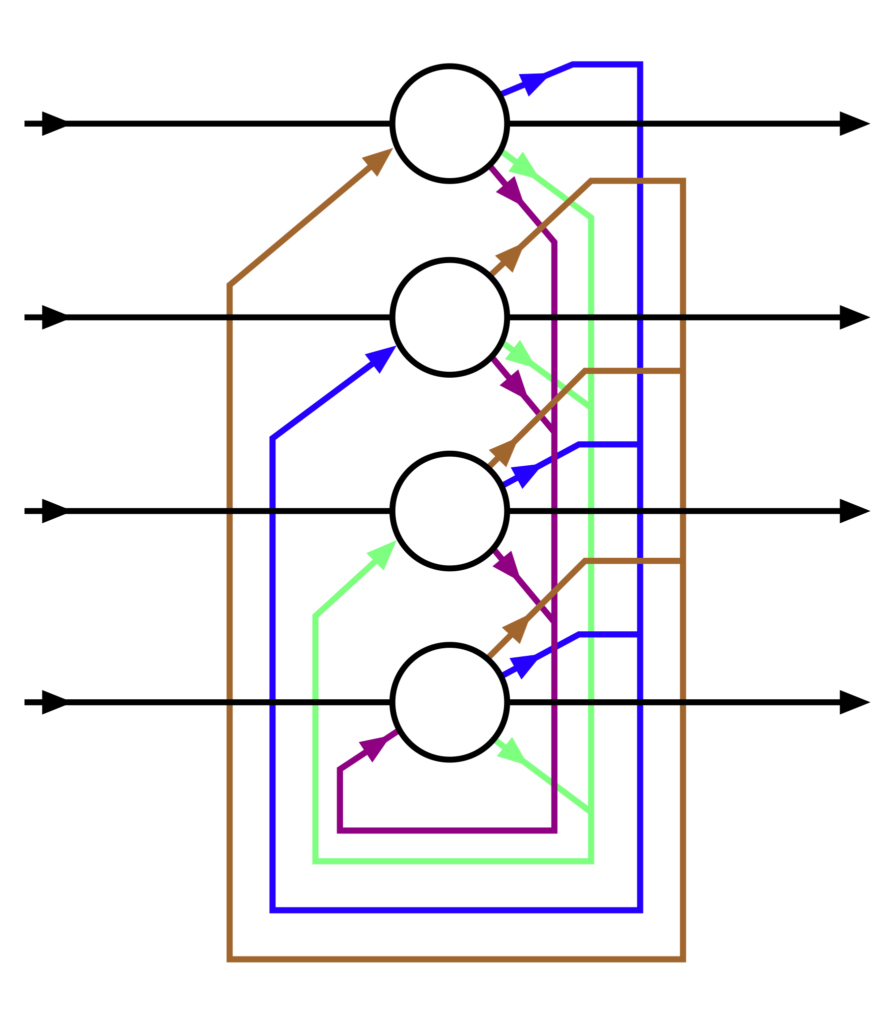
An example of a Hopfield network with four nodes. Via Zawersh on English Wikipedia (CC BY-SA 3.0).
I attended Caltech in the CNS program’s second year. The first words I heard in a classroom were from Hopfield: roughly, “If you can explain how you do something, say solve an equation, odds are computers can already do it better. If you have no idea how you do it, say how you recognize your mother’s voice, odds are we have no idea how it works, and computers can’t get close.” That insight explains, among other things, why computers are better at following rules than at making sense of real life.
Hopfield himself was imposing, in a grandfatherly way. Six feet tall, he seemed even taller when tipping back on his heels, clasping his hands, looking benignly down his nose and speaking in a booming bass voice. When graduate student Mike Vanier performed an imitation of Hopfield in a skit during our first year, it brought down the house.
That core class Hopfield taught on Neural Nets (in 1988) was difficult in an epic way. Homework for the very first week — the same week students were still getting computer accounts and finding the bookstore — involved a set of three different kinds of supremely hard problems: solving a difficult set of differential equations, writing and testing a computer program to simulate a simple neural circuit and constructing that same working “neural” circuit by wiring a battery, op-amps, resistors and capacitors up to blink LEDs. Neither programming nor soldering was a stated class requirement. Lucky for me, I already knew how to program and build circuits, so I passed.
In fact, the little circuit I built in Hopfield’s class proved to be a key to my PhD project, and key to his final question for me. Hopfield sat on my committee and approved my thesis — even though I had proved that real neurons can’t possibly operate like those circuits. (Real neurons have to be hundreds-fold faster, at least.)
An interdisciplinary paradise
By world standards, Caltech is a tiny and very exclusive university, having only about a thousand undergrad and another thousand graduate students. Caltech specializes in leading scientific trends; the new CNS program (started with silicon guru Carver Mead) was meant to create an entirely new field by using mathematical techniques from physics, electronics and computer science to understand how information moves in biological systems like brains, muscles, eyes and ears.
Psychology, psychophysics, optics, silicon design, algorithms, neuroscience, robotics — a score of scientific disciplines overlapped in lecture halls, classrooms and labs. It was an interdisciplinary paradise. Caltech’s philosophy is to base nearly everything (even biology) on physics principles. Hopfield and Mead’s common treatment of biological information processing as continuous equation accessible to physics, following in this tradition, made the CNS program a scientific innovation.
Hopfield’s contribution to physics, and to science in general, was to link well-established math about molecules and crystals to poorly understood computation problems like parallel processing and machine intelligence. His key scientific invention (the Hopfield network) was complex enough to solve real and interesting AI-like problems but simple enough to explain through equations initially designed to describe crystal formation. Hopfield created a whole new form of analog computation with his nets and a whole new way of describing neurons with the math behind them.
(While they make the math easier, it turns out that Hopfield’s smoothly responding mathematical “neurons” are nothing like real neurons in brains. Real neurons make irregular pulses whose noisy crackle must, in fact, carry information — a point first made in my dissertation under Christof Koch. [You can read the PDF here.] This fact undermines the one thing neuroscience thought it knew about the neural code.)
The Caltech CNS program was a university-wide expansion of Hopfield’s approach, bringing together math-wielding theorists with lab-bench experimentalists. As a member of both camps, I was in my element, and everyone around me was, too. It was exhilarating to bring humanity’s last 50 years of technological progress in audio, radio, circuits and computers to bear on explaining how brains work and what they do. With CNS, Hopfield and Mead had built a whole new discipline around their visions of mathematically simple neural nets.
I benefitted directly from a major initiative of Hopfield’s. While he was on my committee, Hopfield wrote to the Caltech faculty at large, advising that he would require any grad student getting a PhD with him (e.g., me) to write a single-author paper. Usually, every paper a grad student writes has their advisor’s name on it too. That meant no one was sure whose ideas were whose. Hopfield’s point was that if a student submits a paper entirely on their own, it proves the ideas are theirs. I don’t know how my advisor responded, but I heard the faculty collectively was in a rage: Junior professors needed those extra papers to fill out their publication lists. Publish-or-perish was very real to them, and Hopfield’s principled stand for intellectual integrity made life tougher.
But not for me. Hopfield had “forced” me to do what I always wanted to do anyway: publish my most radical ideas as clearly as possible, in my own voice. So, I wrote a paper pretty much proving that neurons could operate a hundred-fold faster (i.e., 10,000% faster) than anyone thought at the time, which means a hundredfold more bandwidth. That paper started my career as a renegade and bandwidth advocate, a lonely position now utterly validated by many lines of evidence showing sub-microsecond processing in brains, as presented in Tucson this April. Thanks to John Hopfield’s principled vision of science, I was not pressured to water down a good clean idea, which has now been vindicated.
A true physicist
The last conversation I remember with John Hopfield was when I defended my Ph.D. dissertation (the one “disproving” his model of neurons) in the old, storied East Bridge building at Caltech.
This room was nearly sacred to physicists. Steven Hawking had answered questions on these tiles a couple of years before. An alcove across the hall displayed a working micro-motor, less than a tenth of one millimeter on a side, inspired by nano-tech founder (and Nobelist) Richard Feynman. Around the corner were (not-yet-Nobelist) Kip Thorne’s framed bets about black holes. In a tiny room just down the hall, their common advisor John Wheeler had derived quantum mechanics from information theory on a chalkboard — “It from Bit.” On the floor in front of me (I had arrived early) sat his former student Kip Thorne.
In this hallowed place, I had not expected more questions. I had already been answering questions for hours in the seminar room next door, and I frankly expected Hopfield to say something different. I expected him to say “Congratulations, Dr. Softky.” This was supposed to be the end of my dissertation exam.
“We’d like to ask you some more questions,” Hopfield told me.
This wasn’t how it was supposed to work. Moments before, during my PhD defense, I had proved a popular body of knowledge wrong by invoking undisputed math. The panel had accepted the debunking, as CNS co-founder Carver Mead had accepted it weeks before. But I hadn’t debunked physics itself; I had debunked neuroscience. To my committee, that was a lower form of science, and they wanted to make sure I actually knew physics.
So, Hopfield asked me a question that hit the heart of my dissertation. He drew a little diagram of a circuit on the chalkboard: a battery, a capacitor, a resistor and a tiny neon bulb. He asked me what it would do.
I remembered that little circuit from my childhood as a relaxation oscillator. It charges up until it hits the voltage where the bulb lights and then dumps the charge, starting the cycle anew. In other words, it goes blink-blink-blink. That little circuit was exactly the model of a neuron that my dissertation had disproven (such a circuit can’t produce the “noisy” pulses that real neurons produce). It was also the one Hopfield had inflicted on his students in our very first week of class, to solve, program and simulate with wires. Now I got to tell him how it worked, and didn’t work, as I became one of his own program’s very first PhDs.
Very few people create whole new forms of science and technology. Hopfield was the first to use laws of physical energy flow to calculate information flow, just like Mead was the first to use laws of physical structure to design integrated circuits.
Combined, those two ideas now let computers act like dumb or clumsy people. Soon, they will also let us know how brilliant, graceful human beings do what we do best.
The views expressed in this article are the author’s own and do not necessarily reflect Fair Observer’s editorial policy.
Support Fair Observer
We rely on your support for our independence, diversity and quality.
For more than 10 years, Fair Observer has been free, fair and independent. No billionaire owns us, no advertisers control us. We are a reader-supported nonprofit. Unlike many other publications, we keep our content free for readers regardless of where they live or whether they can afford to pay. We have no paywalls and no ads.
In the post-truth era of fake news, echo chambers and filter bubbles, we publish a plurality of perspectives from around the world. Anyone can publish with us, but everyone goes through a rigorous editorial process. So, you get fact-checked, well-reasoned content instead of noise.
We publish 2,500+ voices from 90+ countries. We also conduct education and training programs
on subjects ranging from digital media and journalism to writing and critical thinking. This
doesn’t come cheap. Servers, editors, trainers and web developers cost
money.
Please consider supporting us on a regular basis as a recurring donor or a
sustaining member.
Will you support FO’s journalism?
We rely on your support for our independence, diversity and quality.
Comment